Predicting Proteins
Jens Meiler
Vanderbilt University and Leipzig University
Published November 25, 2024
Jens Meiler was only 12 years old when he learned programming on a computer smuggled back to East Germany. It was 1985, a few years before the wall came down, and his father had sneaked the device across the border from Austria. “My dad brought back a computer, and my mom taught me how to program the machine,” Meiler remembers.
In high school, after the reunification of Germany, Meiler’s skills advanced on neural networks programmed by other scientists. By the time he became a chemistry undergraduate, he was proficient enough to design his own from scratch. “That neural network was used to predict small molecule properties—it absolutely shaped my career,” he says.
Fast forward to today, and Meiler is an experienced hand at what most of the world celebrates as new technology—using the tools of artificial intelligence (AI), specifically artificial neural networks—to predict how proteins fold and function and to discover new drugs.
He now heads two large laboratories—one at Vanderbilt University in Nashville, the other at Leipzig University in Germany—where his teams are developing algorithms that could help design therapies for a staggering range of diseases, such as cancer, cystic fibrosis, viral infections, or neurological diseases.
“This is not a new thing,” Meiler says. “We have been developing and using these technologies for 30 years. What changed is the computers got much faster and data sets got much larger. As a result, one can train artificial neural networks in a way that’s much more complex.”
Early Immersion
Meiler’s interest in chemistry and computation was sparked early by his parents, both scientists. His father, a physicist, and his mother, a mathematician, supported his natural curiosity and love for science. He grew up in Leipzig when it was part of the Soviet-controlled Eastern Bloc.
After the Berlin Wall fell, he stayed close to home to pursue a chemistry degree at the University of Leipzig. There, he was inspired by early reports of using neural networks to predict protein structure. The neural network algorithm he created fueled dozens of papers and projects that followed in his laboratory and that still forms the basis for the evolving capabilities of protein structure and prediction.
For his PhD, Meiler chose the nuclear magnetic resonance spectroscopy laboratory of Christian Griesinger at University of Frankfurt. But even as he delved into experimental science, his mind was always drawn back to computation.
This dual interest in structural biology and computation took him to Seattle to work with David Baker, a winner of the 2024 Nobel Prize in Chemistry for computational protein design. Baker made an immediate impression when Meiler was deciding among U.S. laboratories for a postdoctoral position.
“When you talk to him, innovation just pours out of him,” he says. Of his interviews, “everyone else asked me where you’re coming from, what you’re doing. He asked, ‘If I wanted to move two amino acids in a protein structure, how can you simplify the mathematical equation so that it is linear?’”
In Baker’s laboratory, Meiler contributed to the development of Rosetta, a software suite that is now a cornerstone in the field of protein structure prediction. Some of the Rosetta code comes from the original neural network he created as an undergraduate. The Meiler laboratory continues to help develop Rosetta software, as well as other tools and also hosts a bi-annual workshop to train academic and industry users on the software.
Integrating Artificial Intelligence with Experimental Data
Meiler’s contributions to Rosetta cemented his place in the scientific community. He had multiple job offers from Germany, but Baker advised him to stay in the United States. He joined the Vanderbilt University faculty in 2005.
The Meiler laboratory now spans two continents. In 2020, he accepted a prestigious Humboldt Professorship at Leipzig University, where he heads an institute dedicated to drug discovery. Between the two laboratories, he manages about 60 scientists and staff, who work in teams toward the same goal: integrating artificial intelligence and deep neural networks with biophysics to tackle some of the most pressing problems in medicine.
As a strategic move in the structure prediction world, Meiler staked out membrane proteins and their dynamics as starting points for small molecule drug discovery. Membrane proteins skulk within the cell wall, where they orchestrate the interaction of the cell with its environment. They account for about 25 percent of the protein-coding genome but more than 50 percent of known drug targets.
The potential medical applications motivate funding agencies to support his intersecting passions of developing new algorithms for protein structure prediction, protein engineering, and drug discovery that keep laboratory members busy on both sides of the Atlantic.
One of the tools that has been instrumental in this research is RosettaLigand, which Meiler first programmed. RosettaLigand allows scientists to model how small molecules bind to proteins, predicting their interactions and providing insights into which compounds are most likely to be effective as drugs.
Another major research area for the lab—designing proteins, specifically antibodies and vaccines—soon arose, enriched by a collaboration with Vanderbilt colleague James Crowe, a leading expert in antibody discovery.
In Leipzig, one of his teams is working on designing nanoparticles loaded with a vaccine or therapeutic agent directly to specific human cells. Meanwhile, in Nashville, Meiler’s group is using AI to identify small molecules that can rescue malfunctioning proteins. “We’ve been screening libraries of small molecules to find drug candidates for diseases like cystic fibrosis that can rescue a mutation,” he says. “There are hundreds of mutations that cause the disease, and we’ve only found drugs for a few.”
From Sequence to
One of the most talked-about recent breakthroughs in protein science is AlphaFold2, an AI system developed by Google’s DeepMind that made headlines for accurately predicting protein structures from the genetic sequence. In fact, AlphaFold2’s architects shared the 2024 Nobel in chemistry. But Meiler is both an admirer and a critic.
“AlphaFold is awesome,” he says. “It just broke the glass ceiling, moving from regular neural networks to deep neural networks and training them the right way.” AlphaFold tapped into the massive experimental data set of the Protein Data Bank of 200,000-plus proteins and their corresponding sequences.
But, he adds, AlphaFold “also ignores biophysical principles, because the hypothesis was that AI can do it all. The correct result is in there, but there are a whole bunch of alternatives, and a portion of these alternatives are biophysically not realistic. They have so much energetic frustration that they will never exist in that way. So we need to reconcile AI with biophysical energy functions. And we need to move both of these things together to be applied to biomedical research and human-relevant disease states. These are the next steps.”
Meiler’s team is working on pushing AlphaFold’s capabilities further by creating more code to use AlphaFold in new ways. “We hacked AlphaFold to make biologically relevant alternative conformations,” he says (del Alamo, Sala et. al, eLife, 2022).
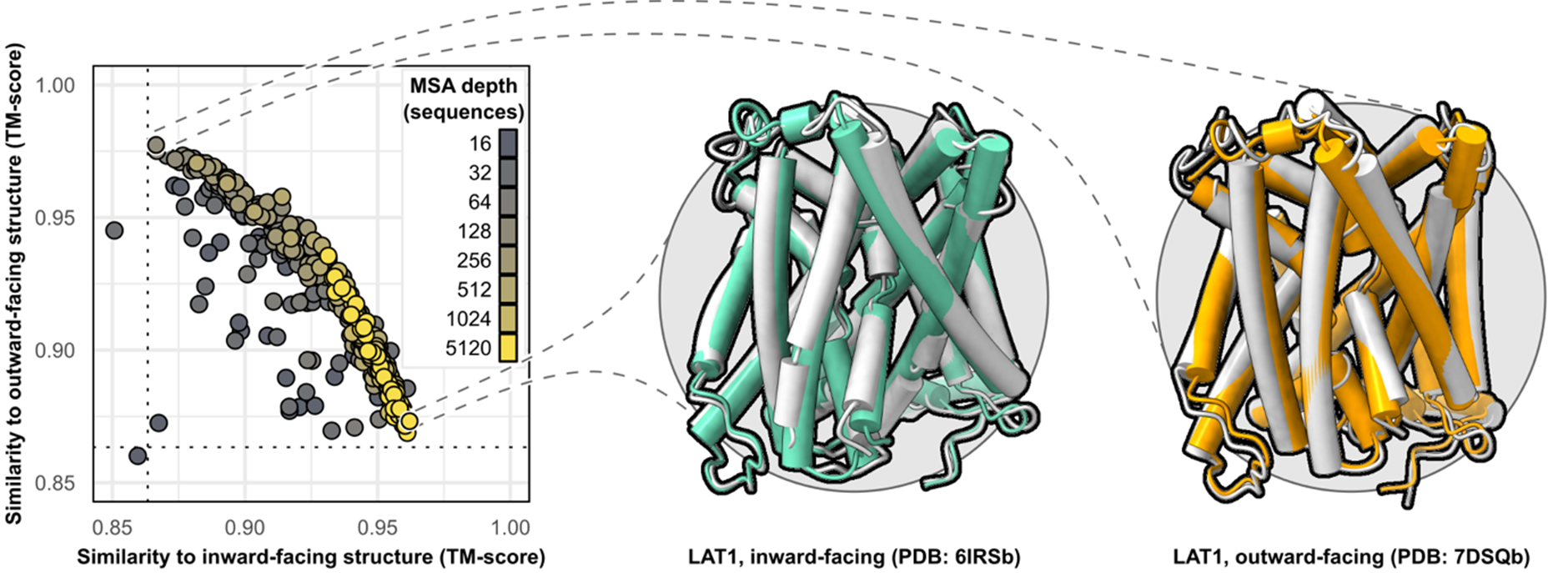
Personalized Structural Biology
These deep machine learning technologies will take over structural biology for a while, Meiler predicts. “I think moving forward using Rosetta as a platform to unite all of these AlphaFold and biophysical technologies into one frame is going to be important,” he says.
He’s particularly focused on using AI to advance what he calls “personalized structural biology,” where therapies are tailored to a patient’s genetic profile. "You have 10,000 mutations in your genome," he says. "With AlphaFold, Rosetta, and all the tools, we can build models of how those mutations might interfere with their action and ultimately find small molecules based on that structural understanding."
The near future may also see a renaissance of neural networks designed for smaller, noisier data sets to achieve a targeted solution, he says. “The expectation is the one will have to be more clever and also more tailored to specific diseases,” he says. “There may not be one model that cures them all.”
Despite his busy schedule—he flies between Leipzig and Nashville about six times a year—Meiler is enthusiastic about the future of his work. “I tell my students, I don’t care which disease you cure, as long as you cure a disease,” he says, laughing. And with his teams on the verge of new discoveries, it seems likely that those cures may be closer than ever.
--Carol Cruzan Morton