Garbage Out
Kay Diederichs
University of Konstanz
Published March 30, 2017
In 2011, Kay Diederichs welcomed longtime friend and colleague Andrew Karplus into his lab at the University of Konstanz in Germany. The two had met in the 1980s at the University of Freiburg when Diederichs was a graduate student learning X-ray crystallography.
Over the years, they’d tried to improve the tools structural biologists use to assess X-ray diffraction data quality. Their efforts were respected; a 1997 Nature Structural Biology paper they co-wrote describing a new data quality indicator has been cited around 800 times. Yet few used the new formula. “People stuck with the old indicator,” says Diederichs. “It was frustrating.”
This time, they decided, things would be different. Instead of focusing on what the formula measures, they focused on the ways in which data quality guidance produces more meaningful results. The output of their efforts in 2011 was CC1/2, an indicator that measures the information in a data set and helps crystallographers choose which data to use or not use. “CC1/2 gives you a sensible answer about whether the data are good for improving the model or not, so then by using the good data, we get better models,” says Diederichs.
The work fits into Diederichs’ career as a structural biologist and methods developer. The current focus of his work is finding ways to apply new methods to multiple modes of data collection, from X-ray diffraction to cryo-electron microscopy, X-ray free electron laser (XFEL) technology, and electron diffraction. “I think the future lies in the combination of methods so we can all learn from each other,” he says.
Diederichs saw his first structure — a 1988 Nobel Prize winning photosynthetic membrane protein structure — as an undergraduate studying biophysics at the University of Freiburg in the 1980s. He immediately decided to pursue structural biology and joined the lab of Freiburg’s Georg E. Schulz. “He took me on as a physicist because at that time, you needed a physicist to solve structures,” says Diederichs.
The primary tool available at the time as mosflm, which was used to process photographic films of X-ray diffraction data. But XDS, written by Wolfgang Kabsch, had also emerged as the first computer program to process digital X-ray data. In XDS, Kabsch had solved and automated the geometrically very difficult indexing problem. “I was probably one of the first XDS users,” says Diederichs. “It was clear from the code that the author was a genius.”
As a graduate student, Diederichs got to know Kabsch, who worked nearby in Heidelberg. The two stayed in contact loosely over the years, and then, in 2007, Diederichs realized that it was getting to be time for Kabsch to retire. Since he knew Kabsch and XDS and FORTRAN, he offered to help maintain XDS as a side project. Kabsch agreed. “It’s been ten years now, and the situation is the same,” says Diederichs, who shares maintenance duties with Kabsch and teaches XDS workshops. “Kabsch is not retired. He’s in the middle of retiring.”
After earning his doctorate from the University of Freiburg, Diederichs joined Karplus’s lab at Cornell for a post-doc in 1990. Even then, says Diederichs, “We were not happy with the situation of statistical indicators in crystallography.”
At the time, there were two indicators. One, the R-factor, had been developed in the 1950s. The other, Rsym, had been developed for a narrow purpose related to a specific detector, but had ended up being adopted widely to assess data quality in general. “The formula got a life of its own,” says Diederichs. “It was misunderstood.”
Diederichs and Karplus identified the weaknesses in Rsym and described them, along with a replacement, in 1997. Then in 2011, they tried to change the status quo again with the development of CC1/2. “Not everyone has converted, but young people have,” says Diederichs.
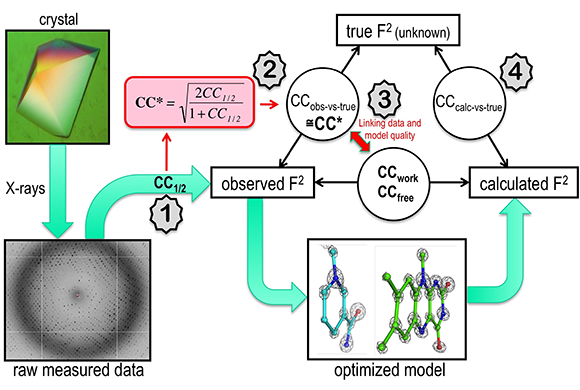
This tool is needed more now than ever. In the past, crystallographers could take years to solve a structure. Today, however, the field is becoming increasingly high-throughput. It is no longer necessary to have a physicist on staff to solve structures because their expertise is now embedded in software. Automating data quality assessments is a natural additional analytical step. “The numbers tell you what to do,” says Diederichs. “Though structural biologists still must understand the indicators.”
Indicators of data quality not only help scientists build better models faster, they also allow researchers to compare data sets to one another and to revisit older data sets and re-assess the models built from them. “This is a rational approach to science rather than an approach that is guided by a particular investigator’s experience,” says Diederichs. “If you have indicators that are meaningful, they can replace experience.”
--Beth Dougherty